The implications, uses, areas and potential of artificial intelligence are unlimited and of a completely different nature from one another and those who are assiduous readers of this blog will have noticed how AI has a significant presence within it . Let us now look at another aspect of this technology which concerns a more media and wide-ranging sphere; if in recent years there has been much talk of fake news, that is to say of deliberately false news, written and disseminated in order to create misinformation, soon we will talk about their qualitative leap, a type of distortion of reality in which it will be easier to fall and which will be more difficult to refute through a classic journalistic investigation and a careful research of the sources: the deepfakes. In a nutshell, they are images or more easily videos, in which anyone thinks of watching a character say or do things, but nothing is true. The first examples of deepfake date back to 2017 when this type of technology was used, in a pornographic context, to spread fake videos starring famous people. These were videos in which the face of an actress or a porn actor was replaced by that of a Hollywood film actor, through a technique called “contradictory generative networks” or GAN. But let’s see more specifically how this is possible; to explain the deepfakes, the thing to be recuperate, perhaps not simpler but certainly more explanatory, is the concept of autoencoder, an artificial intelligence system built on several neural layers that manages to decode and encode an input or signal. The autoencoder, starting from an image, a symbol or a sequence constructs a representation, a mask, which interprets as a reference to the initial content. This process can be used, for example, in the case of ruined or deteriorated contents or symbols: in fact the autocoder will be able to reproduce the damaged part, reconstructing the missing part and thus improving its yield. If we take the specific example of a human face, the autoencoder, starting from the original face, will create a so-called latent face, or base vector, and then return it, through a decoder, reconstructed or improved. Here, in the concept of deepfake, everything acquires a different meaning. In this case in fact encoder and decoder are divided, decoupled; on one side we will therefore have a coder which from face A finds the latent face A, but on which another decoder, that of B, is applied, thus reconstructing the facial part of A on B. To have a more comprehensible view of these passages which we refer to image 1:
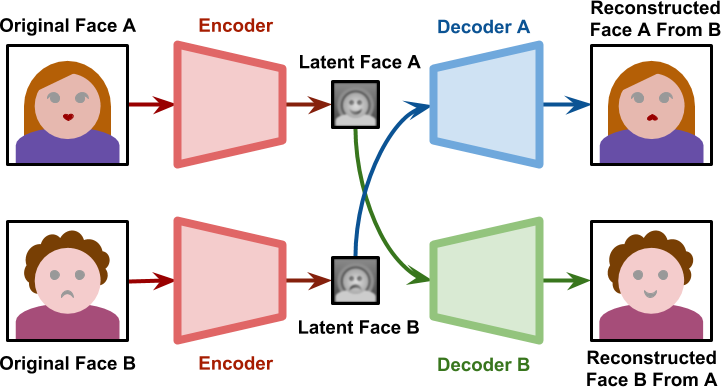
As one can easily imagine, this opens the doors of imagination and the cases of application are infinite. But what impact can such a potential use of technology have on society around us? In a narrative in which a subject is visually convinced that something is happening even if it is not, what precautions or solutions can be adopted?
Since the deepfakes appeared on the internet, the researchers immediately started studying methods to unmask fake videos. One of these was based on the identification and recognition of small gestures, imperceptible signs of the body, such as the frequency with which the eyelids are shaken. But unfortunately even the latest generation of deepfake has immediately adapted to this mechanism, forcing research to find new solutions. Other methods to unmask deepfakes are the analysis and observation, using an algorithm, of the pixels in some specific frames. Another use of pixels, this time for precautionary purposes, involves the insertion of some of these in the background of an image as a disturbance in order to prevent future manipulations.
Unfortunately, what is still certain today is that the “fakers” are always at the forefront and that at the moment there are no real solutions, but more than anything a continuous race towards remedies.
Recent Comments